Revolutionizing Information Retrieval: A Deep Dive into AI Search Technologies
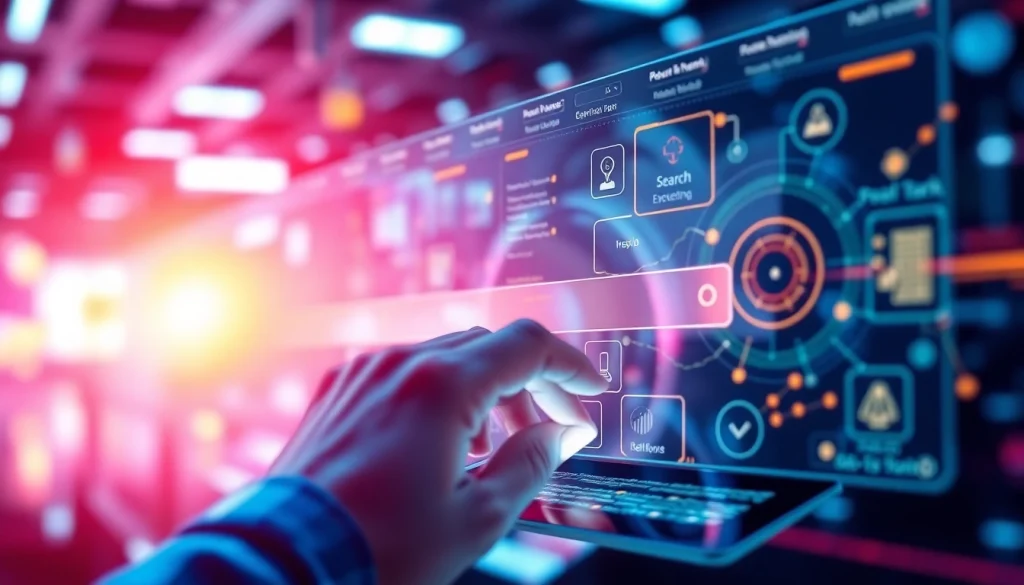
Introduction to AI Search
The rapid evolution of technology has profoundly transformed the way we interact with information. At the forefront of this transformation is AI search, a groundbreaking approach that leverages artificial intelligence to enhance the search experience. Unlike traditional search engines, AI search utilizes advanced algorithms and machine learning techniques to understand user intent, deliver contextually relevant results, and improve overall accuracy. In this comprehensive article, we will explore the nuances of AI search technologies, their features, challenges, and what the future holds for this dynamic field.
What is AI Search?
AI search refers to the implementation of artificial intelligence techniques within search engines to provide enhanced data retrieval capabilities. At its core, AI search functions by understanding natural language queries and delivering results that are not only relevant but also aligned with the user’s intent. This is achieved through complex algorithms that analyze vast amounts of data to discern patterns, context, and relevancy in search queries.
The Evolution of Search Technologies
The journey of search technologies began with simple keyword matching, which has progressed to more sophisticated systems capable of semantic understanding. Early search engines operated largely on Boolean logic, which required users to formulate queries using specific operators. However, as the internet expanded, the limitations of such systems became apparent. Innovations like Google’s PageRank algorithm marked a significant leap forward by introducing a value-based approach to ranking web pages, setting the stage for advanced AI search technologies.
The introduction of machine learning and natural language processing (NLP) in the last decade has revolutionized search capabilities. Modern AI search engines, such as Google’s Gemini and Microsoft’s Copilot, utilize NLP to interpret user queries more effectively, contextually matching search results beyond simple keyword recognition.
The Importance of AI in Modern Search
AI plays a critical role in modern search engines, enhancing user experience by making searches more intuitive and efficient. Here are several key benefits of incorporating AI in search:
- Personalization: AI algorithms analyze user behavior and preferences to tailor search results, creating a more personalized experience.
- Contextual Understanding: With advancements in NLP, AI search can understand the meaning behind queries, providing results that consider context rather than just keywords.
- Speed and Efficiency: AI enhances the processing speed of search engines, allowing them to deliver results in real-time, even for complex queries.
- Reduced Ambiguity: AI helps in clarifying ambiguous queries by asking follow-up questions or presenting different interpretations, ensuring that users find exactly what they seek.
Key Features of AI Search Engines
Understanding Natural Language Processing
Natural Language Processing (NLP) is integral to AI search. It enables machines to interpret and manipulate human language, facilitating a more natural interaction between the user and the technology. NLP breaks down language into simpler components, allowing AI systems to analyze various aspects such as syntax, semantics, and sentiment.
AI search engines utilize NLP techniques to dissect user queries, recognizing nuances in language that can significantly affect search results. For instance, the phrase “Apple” could refer to either the fruit or the technology company based on context. An effective AI search engine will interpret this context and provide results accordingly.
Integration of Machine Learning Techniques
Machine learning, a subset of AI, plays a crucial role in improving search algorithms. By continuously learning from user interactions, AI-driven search engines can adapt, evolve, and become increasingly effective over time. Machine learning techniques, such as supervised learning and reinforcement learning, help these systems to:
- Refine their understanding of user intent based on past behavior.
- Improve ranking algorithms through feedback loops that consider which search results users select.
- Generate predictive models to anticipate user needs based on contextual clues.
For example, if a user consistently searches for travel-related information, a machine learning-powered search engine may prioritize tourism-related results if they search for “Los Angeles,” understanding the user’s intent more accurately.
User-Centric Design and Experience
Designing AI search engines involves a user-centric approach, ensuring that the search experience is not only efficient but also enjoyable. Features like voice search, personalized recommendations, and interactive search results contribute to a holistic user experience. AI search engines tend to present information in easily digestible formats, such as snippets or visual content, enhancing user engagement.
Moreover, user feedback mechanisms allow individuals to rate the relevance of search results, contributing to the continuous learning loop that improves the algorithms further. The focus on user experience helps in minimizing frustration and increasing satisfaction with the search process.
Comparative Analysis of Leading AI Search Platforms
Overview of Top Contenders
As the market for AI search engines grows, several platforms have emerged as frontrunners. Notable players include:
- Google Gemini: Google’s latest offering merges search capabilities with AI chatbots, providing context-aware responses that enhance user interaction.
- Microsoft Copilot: This platform integrates AI-powered suggestions into standard workflows, streamlining information processing and retrieval.
- ChatGPT Search: By harnessing GPT-4’s capabilities, ChatGPT Search delivers quick and relevant answers with contextual awareness, making it a preferred choice for many users.
- Perplexity: Focused on user questions, Perplexity feeds user input into its algorithms to deliver tailored search results effectively.
Strengths and Weaknesses
While each platform offers unique advantages, they also come with their limitations:
Google Gemini:
Strengths: Extensive data coverage, powerful algorithms for context interpretation, and integration with existing Google services. Weaknesses: Potential biases in search results based on popular trends rather than individualized needs.
Microsoft Copilot:
Strengths: Seamless integration into Microsoft Office applications, enhancing productivity and decision-making. Weaknesses: Limited to the Microsoft environment, which may restrict broader functionality compared to standalone search engines.
ChatGPT Search:
Strengths: Immediate responses and strong conversational abilities. Weaknesses: Limited ability to handle complex queries that require extensive contextual knowledge.
Perplexity:
Strengths: Focused on delivering direct answers to user questions. Weaknesses: May lack in-depth analysis for broader context.
Use Cases and Applications
AI search engines are versatile tools that cater to various use cases across different sectors:
- Content Creation: AI search helps content creators find relevant information quickly, enabling them to produce high-quality outputs.
- Academic Research: Researchers can leverage AI search to access papers, articles, and studies with enhanced accuracy and context.
- Customer Support: Chatbots equipped with AI search capabilities can deliver immediate answers to common queries, enhancing customer satisfaction.
- Data Retrieval in Business: Organizations utilize AI search to quickly fetch business intelligence and insights from vast data sets, improving decision-making processes.
Challenges and Limitations of AI Search
The Citation and Accuracy Issues
While the advancements in AI search technology are significant, challenges persist, particularly regarding citation accuracy. AI systems often struggle to track the source of information, which can lead to a lack of accountability regarding the accuracy of content. For instance, research conducted by Columbia Journalism Review highlighted that many AI search engines struggle with properly citing news sources.
Ensuring that AI search systems provide reliable sources and citations remains a critical challenge. This necessitates the implementation of solutions that can accurately trace back content origins.
User Privacy Concerns
As AI search engines improve personalization through data analysis, they also raise critical privacy concerns. Users often have reservations about how their data is collected, used, and stored. Transparency in data practices is essential, as users become increasingly cautious about sharing their information.
Effective solutions include implementing robust data protection policies and providing users with control over their data preferences, enhancing trust while still delivering personalized search experiences.
Technical Barriers and Future Solutions
Technical challenges also impede the growth of AI search, including the need for continual data training, algorithmic updates, and maintaining infrastructure to support advanced AI capabilities. These issues often require significant resources and expertise.
To overcome these hurdles, organizations can invest in training and intuition-building programs for AI models and collaborate with tech specialists to ensure smooth operation and updates. Furthermore, strategies for sustained real-time feedback can enable continuous learning and improvement of AI algorithms.
The Future of AI Search Technology
Emerging Trends in AI Search
The landscape of AI search is poised for further evolution, with several trends shaping its trajectory:
- Increased Multimodality: Future AI search engines may evolve to interpret data that integrates text, images, and videos, creating a more enriched search experience.
- Conversational Searches: The shift toward conversational interfaces will redefine how users interact with search engines, enhancing accessibility and usability.
- Enhanced Predictive Capabilities: As machine learning techniques advance, AI search engines will become better at anticipating user queries based on previous interactions and context.
Potential Advances in User Experience
Advancements in AI search are likely to emphasize user experience even more. Innovations may include:
- Voice-Activated Searches: As voice recognition technology improves, users may engage with AI search through voice queries more frequently.
- Augmented Reality (AR) Integrations: The integration of AR could allow users to interact with digital information in their physical environment, transforming how search results are explored and utilized.
- Adaptive Learning Systems: AI search engines of the future may dynamically adjust to user preferences and trends, refining their outputs increasingly tailored to individual behaviors.
Predictions for the Next Decade
As we look toward the next decade, AI search technology is set to revolutionize information retrieval further. Predictions for AI search include:
- Widespread adoption of integrated AI search across various platforms, enhancing operational efficiencies.
- The emergence of decentralized search systems that empower users with greater control over data privacy while still leveraging AI capabilities.
- Continued investments in ethical AI practices, ensuring that emerging technologies align with user privacy and accuracy standards.
In conclusion, AI search systems are transforming the landscape of information retrieval, offering users more intuitive and effective ways to engage with data. By understanding their capabilities, challenges, and the future trends that will shape them, stakeholders can better prepare for a world where searching for information becomes an increasingly seamless and advanced experience.