How AI Search Engines Are Reshaping Online Discovery and Information Retrieval
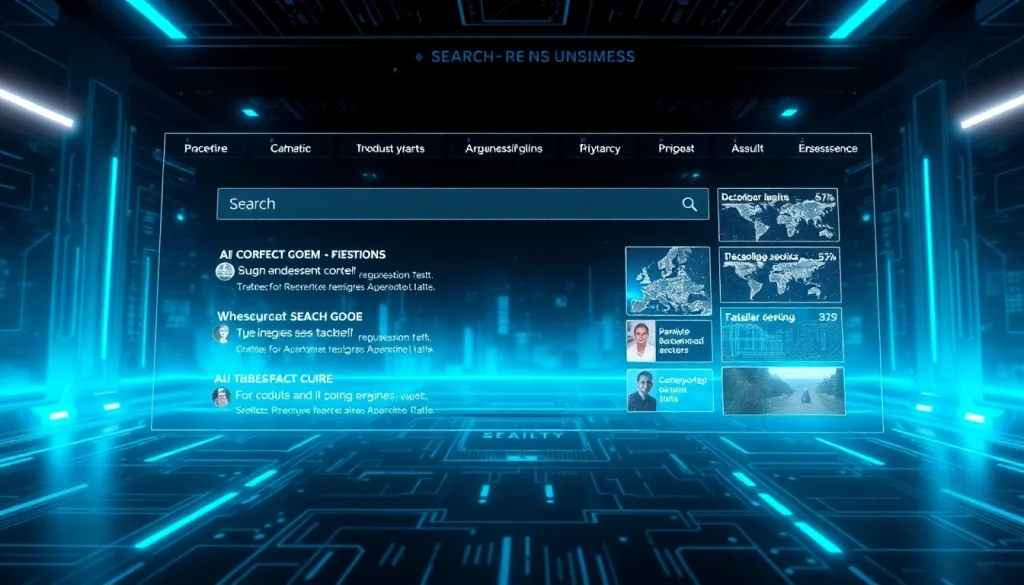
Understanding AI Search Engines
In the digital age, information is abundant, and the ability to efficiently access relevant content has become paramount. Traditional search engines like Google have paved the way for rapid information retrieval, but as technology evolves, so do our tools for searching the web. Enter the AI search engine, a revolutionary advancement that combines artificial intelligence with search functionalities to enhance the user experience significantly. This article delves deep into what AI search engines are, how they reshape our information retrieval processes, and what the future holds for this rapidly growing field.
What is an AI Search Engine?
An AI search engine is a software application designed to search for information on the internet that utilizes artificial intelligence technologies to improve the relevance and accuracy of search outputs. Unlike traditional search engines that rely heavily on keyword matching and ranking systems, AI search engines leverage machine learning and natural language processing (NLP) to understand user intent and context more effectively.
The core capabilities of AI search engines include:
- Understanding Natural Language: AI search engines employ NLP to process queries as human language rather than mere strings of characters, allowing them to comprehend complex phrases and sentence structures.
- Learning from User Behavior: With machine learning algorithms, these engines continuously learn from user interactions to refine their results over time, leading to increasingly personalized outcomes.
- Providing Contextual Search Results: AI search engines provide results that are not only relevant but also contextual, taking into account the individual user’s previous searches, preferences, and even their geographic location.
How AI Enhances Search Results
AI-enhanced methodologies comprising various capabilities allow search engines to deliver superior results:
- Semantic Search: By grasping the meaning behind a query, AI search engines connect users with the information they seek through synonyms and related topics.
- Image and Video Recognition: AI can analyze content in images and videos, providing relevant results based on visual data, which standard searches fail to capture.
- Chatbot Integration: Some AI search engines incorporate chatbots, guiding users through their searches interactively, offering suggestions based on context, and enhancing engagement.
Comparison with Traditional Search Engines
To understand the distinct advantages of AI search engines, one must compare them with traditional models. While traditional search engines are effective for straightforward queries, they falter with nuanced questions and complex search intents. Here’s a closer look:
Aspect | Traditional Search Engines | AI Search Engines |
---|---|---|
User Intent Understanding | Limited, mostly keyword-focused | Deep understanding of semantics and context |
Adaptability | Static algorithms requiring manual updates | Self-learning algorithms adapting in real-time |
Search Result Personalization | Generic results based on broad metrics | Highly personalized results based on user data |
Information Format | Text-based results only | Multi-format results including visuals and context |
The Technology Behind AI Search Engines
Machine Learning Algorithms
Machine learning, a subset of artificial intelligence, plays a critical role in the functionality of AI search engines. Through various algorithms, these machines can analyze vast datasets, learn from them, and apply that knowledge to refine search output. Popular machine learning techniques utilized include:
- Supervised Learning: In which the model is trained on a labeled dataset, allowing it to predict outputs based on the input data.
- Unsupervised Learning: Where the model learns patterns in the data without prior labeling, often leading to cluster formation.
- Reinforcement Learning: Involving an agent that learns to make decisions by receiving feedback from its actions through rewards or penalties.
Natural Language Processing Capabilities
Natural language processing (NLP) enables AI search engines to interpret user queries accurately, understanding not only the words but also the underlying intent. Key NLP capabilities include:
- Tokenization: Splitting text into individual words or phrases to analyze grammatical structure.
- Named Entity Recognition: Identifying entities such as names, dates, and locations within a text to enhance search relevance.
- Sentiment Analysis: Gauging the emotional tone of users’ queries to better align results with user sentiment.
Data Sources and Real-time Updates
AI search engines rely on diverse data sources to craft their responses. This can include structured data from databases, unstructured data from web pages, and content from social media platforms. The capability for real-time updates is crucial:
- Continuous Learning: AI search engines leverage real-time data to update their algorithms and improve accuracy.
- Integration of APIs: Many AI search engines connect with external data sources, allowing them to integrate new information instantly.
Benefits of Using AI Search Engines
Personalized Search Experiences
AI search engines are uniquely positioned to customize user experiences. They monitor user behavior over time, adjusting search results to suit individual preferences. This personalized approach is particularly valuable in:
- E-commerce: AI search engines can suggest products based on past purchases and browsing history.
- Content Platforms: Users receive suggestions for articles and videos aligned with their interests.
- Educational Tools: Students can receive tailored resources and answers based on their learning progress.
Improved Accuracy and Relevance
One of the most significant advantages of AI search engines is their ability to deliver more precise and relevant results. This stems from their capacity to understand context and intent, leading to:
- Less Noise: Users encounter fewer irrelevant results, making information retrieval smoother.
- High-Quality Content: AI algorithms often prioritize content that meets higher quality standards, such as credible sources and engaging visuals.
Time-saving Features
Time efficiency is a crucial benefit that AI search engines offer. Features that enhance this include:
- Voice Search: Allowing users to query verbally, speeding up the search process.
- Quick Answers: Techniques like featured snippets provide direct answers at the top of search results, minimizing the time spent browsing.
- Aggregated Data: Delivering summarized insights from multiple sources at once, reducing the need for individual searches.
Challenges and Limitations
Common Issues in AI Search Engines
While AI search engines offer extraordinary benefits, they are not without their challenges. Common issues include:
- Data Quality: Poor-quality data directly impacts the effectiveness of AI systems. Algorithms trained on unreliable data may yield subpar results.
- Over-reliance on AI: Users may become overly dependent on AI algorithms, potentially stunting critical thinking and research skills.
- System Vulnerabilities: AI search engines can be susceptible to hacking and misinformation if not adequately secured.
Addressing Bias in Search Responses
Biases in AI systems present a significant challenge, as these can influence the information delivered to users. Addressing bias requires:
- Diverse Training Datasets: Ensuring training datasets reflect a wide variety of perspectives and demographic groups.
- Regular Audits: Conducting ongoing assessments of algorithms to monitor and mitigate biases in search results.
- Stakeholder Engagement: Collaborating with diverse stakeholders when designing and updating search algorithms.
Privacy Concerns with AI Technologies
With the increased ability to personalize searches, there are growing concerns regarding user privacy. Key aspects to address include:
- Data Collection Transparency: Users must be informed about how their data is collected, used, and stored.
- Implementing GDPR Guidelines: Compliance with regulations ensures user privacy is a priority in AI search engine development.
- User Control: Allowing users to manage their data settings and opt-out of data collection enhances trust.
Future of AI Search Engines
Emerging Trends in AI Search Technology
The landscape of AI search engines is continually evolving. Some emerging trends to watch include:
- Integration of Visual and Voice Search: As technology improves, users will increasingly expect seamless interactions through visuals and voice commands.
- AI-driven Predictive Search: Future AI search engines may predict user queries based on past behavior, further streamlining results.
- Conversational Interfaces: Enhanced chatbots may provide even more intuitive search experiences, allowing for dynamic interactions.
Potential Impact on Digital Marketing Strategies
As AI search engines gain dominance, businesses will need to adapt their digital marketing tactics. This includes:
- SEO Shift: Traditional SEO practices will evolve, necessitating a focus on content that meets AI understanding capabilities.
- Analytics Integration: Companies will need to utilize data analytics to gain insights into how AI engines assess their content.
- Emphasizing User Engagement: Content strategies will need to focus on fostering user engagement and interaction to remain relevant in AI search results.
Exploring New Use Cases and Applications
AI search engines are also positioning themselves for diverse applications beyond conventional web searches. Potential use cases include:
- Healthcare: AI search engines can assist in retrieving medical records and research articles to aid healthcare professionals in decision-making.
- Education: Facilitate personalized learning by providing tailored resources and learning paths to students.
- Corporate Knowledge Management: Businesses can utilize AI search to enhance internal databases, making important documents quickly accessible.